TinyML + Local LLMs: A Trendy Architecture for Efficient and Affordable Edge AI
By Shuyang Zhou 12 months agoChallenge
In recent days, the capabilities of large language models (LLMs) are advancing rapidly, and we are seeing a clear trend in the IoT world where hardware systems invoke these large models to infer more complex data and scenarios.
Then, the next topic of discussion should be – how can this be made more cheaper and efficient?
- How can it be made cheaper? Frequent call and long-term use of large models is expensive;
- Can the waiting time be reduced? The time it takes from sending data to a large model to receiving the inference results can take about 10-40 seconds.
Two Innovative Solutions
1. TinyML as a trigger mechanism for activating large models
- Keyframe Filtering: Instead of constantly feeding data to the LLM, a tinyML model can be processed on hardware device to identify key frames or critical data points from the input stream. These key frames might be images, a snippet of audio, or significant fluctuations from a let’s say – 3-axis accelerometer. Only these selected data points are forwarded to the large model for in-depth analysis, effectively prioritizing important data and eliminating unnecessary processing.
- Reduced Token Usage: By focusing on key frames, the number of tokens sent to the LLM is minimized, leading to significant cost savings. This approach also accelerates the overall response time by concentrating on essential data.
Practical Demonstration at Nvidia GTC conference
We demonstrated the effectiveness of this approach in a TinyML+LLaVA (Local Language Model-based Video Analytics) demo at GTC. We showcased two device combinations:
- Setup 1: a USB camera + Nvidia Jetson Orin AGX
In this standard system, a USB camera was directly connected to an AGX running the LLaVA, continuously processing every captured frame. - Setup 2: SenseCAP Watcher + Nvidia Jetson Orin AGX
This system utilized a TinyML vision sensor that only triggered the LLaVA analysis when the sensor detected a person, thus avoiding non-relevant frames like cats.
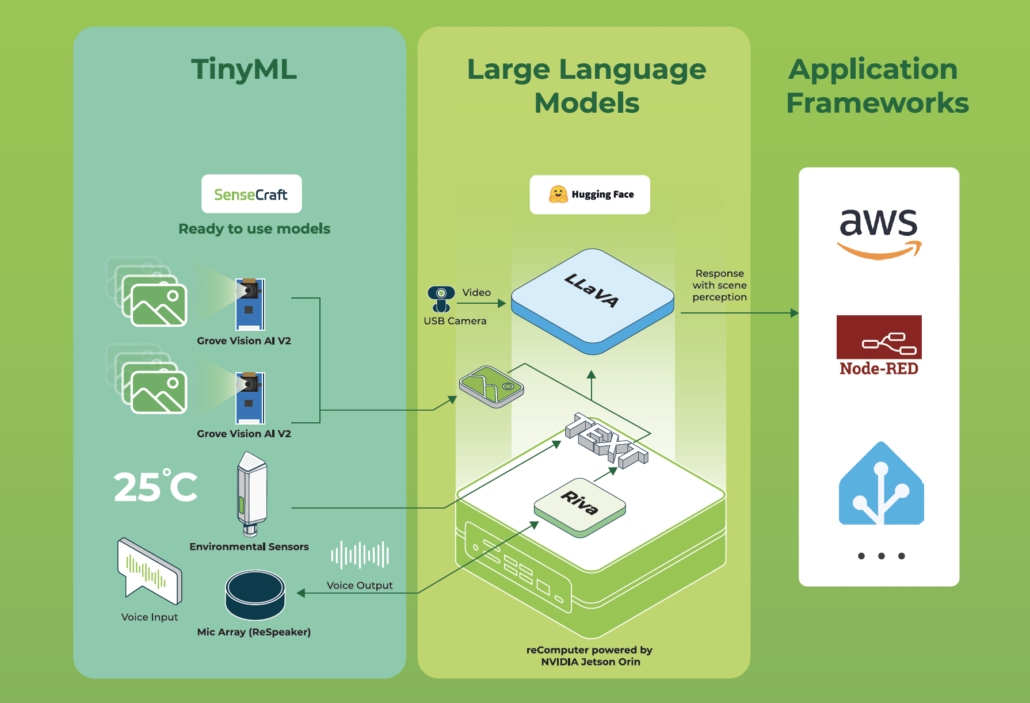
The TinyML configuration demonstrated significant reductions in CPU, RAM, bandwidth, GPU usage, and power consumption compared to the direct LLM call setup.
Here is the demo video.
2. Localization of LLMs on on-premise hardwares
Another approach to optimize cost and reduce latency is to run LLMs on local PCs or embedded computers – which is on-device processing. This approach offers several benefits:
- Cost reduction: Eliminates the bandwidth and API usage fees associated with data transfer and calling remote online LLMs in the cloud.
- Low latency: The latency of obtaining results from LLMs consists of network delays and inference time. By using local LLMs, network latency is minimized. To further decrease inference time, opting for more powerful computers, such as the Jetson Orin AGX, can help achieve even faster response times, potentially as low as 3 seconds.
- Enhanced privacy: Running LLMs locally ensures that your data is not shared with public AI platforms, giving you ownership and control over your data.
Many large models now support local deployment, such as Llama, LLaVA, and Whisper.
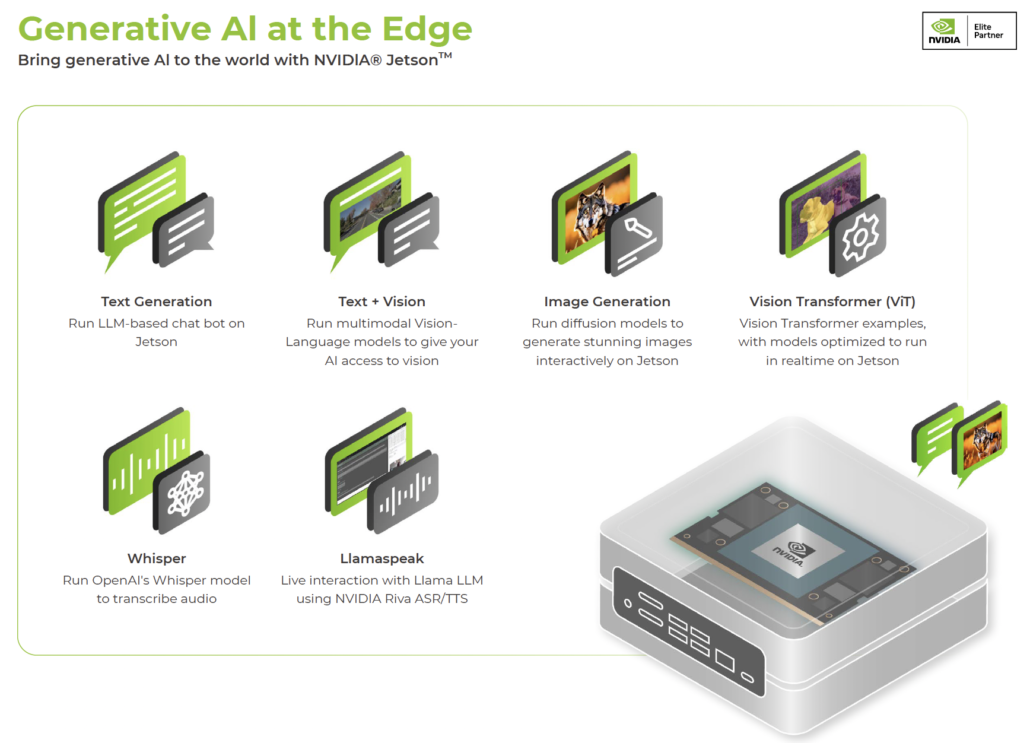
Cost Comparison: GPT-4 Turbo vs Local LLaVA
Then when should you opt for an online large model, and when is it better to go with a local setup?
We conducted a simple cost comparison between the OpenAI’s GPT-4 Turbo API and a local LLaVA setup on a reComputer J4012 in this case.
Each system processed an image sized 640px x 480px once per minute. We aimed to determine when the cumulative cost of using the GPT-4 Turbo API would exceed the one-time purchase price of the local LLaVA setup on the reComputer J4012.
Cost details:
- OpenAI API: $0.005 per image analysis (640×480 px) from OpenAI’s pricing info.
- Local LLaVA: $899 for the reComputer J4012 – NVIDIA Jetson Orin NX 16GB module (one-time cost). Since it operates within a local network, there are no bandwidth costs or API usage fees, making the only expense the purchase of the J4012 device.
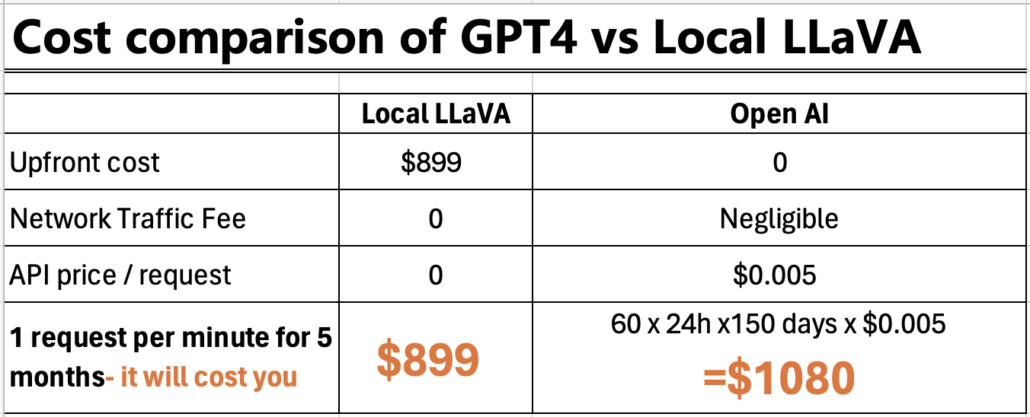
Based on this rough calculation, using GPT-4 Turbo for five months would cost around $1080, which already exceeds the price of J4012.
Therefore, if you only need LLMs for a short term or infrequent usage, opting for a public LLM is suitable.
However, if you require long-term use of them and are sensitive to costs, installing them on a local computer is a very effective strategy. Higher-resolution images incur higher costs, making the cost advantage of local deployment even more pronounced.
But how does the inference speed of localized LLMs?
Please refer to the chart below, where we have quantified various popular Llama models and then tested them on the Jetson Orin AGX 64GB to compare their inference speeds.
If we take human reading speed as a benchmark, which is typically between 3-7 tokens per second, then an inference speed exceeding 8 tokens per second would be quite sufficient. It appears that running a large 13B model is feasible on this hardware, and for a 7B model, the performance is even more superior.
However, if you need to run even larger models, such as a 33B model, and have specific requirements for inference speed, it is advisable to opt for a more powerful computer.
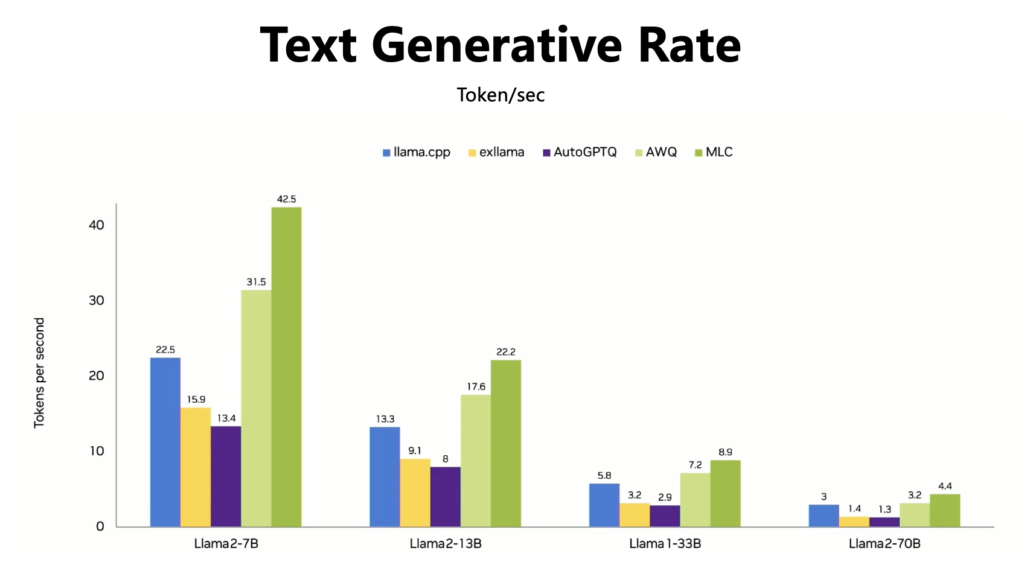
Exploring Seeed's Products Supporting TinyML + Local Generative AI Architecture
Seeed products are divided into two main categories: AI Sensors and Edge Computers.
These products are supported by the Seeed SenseCraft software suites, enabling sophisticated and integrated solutions.
AI Sensors
The representative products of AI sensors are SenseCAP Watcher, Grove Vision AI Sensor V2, and XIAO.
SenseCAP Watcher – a physical LLM agent for smarter spaces. It natively supports various tinyML and Gen AI models. It can assist in monitoring a designated space, detect any activity that matters to you, and notify you promptly on the SenseCraft APP or your own app.
As the world’s first physical LLM agent for smarter space. SenseCAP Watcher is able to:
- Monitor a designated space.
- Identify and interact with targets you specified.
- Spot noteworthy events and give notifications.
Simply give voice / APP commands like “tell me when you see a person,” and the Watcher will notify you when such events occur. But it is not just about detecting targets, it leverages LLM’s capabilities to analyze behaviors and states. Like identifying a person + wearing a red shirt, a dog is tearing up tissues.
Now this product is LIVE on Kickstarter. Click here to back us, and get the early bird price now.
The Grove Vision AI Sensor V2 – is your BEST choice if you want to add a dedicated sub-processor for vector data to your main controller like Arduino / Raspberry Pi. Featuring the Arm Cortex-M55 & Ethos-U55, it provides a 480x uplift in ML performance over existing Cortex-M based systems.
Or build your own AI sensors with XIAO – tinyML MCUs.
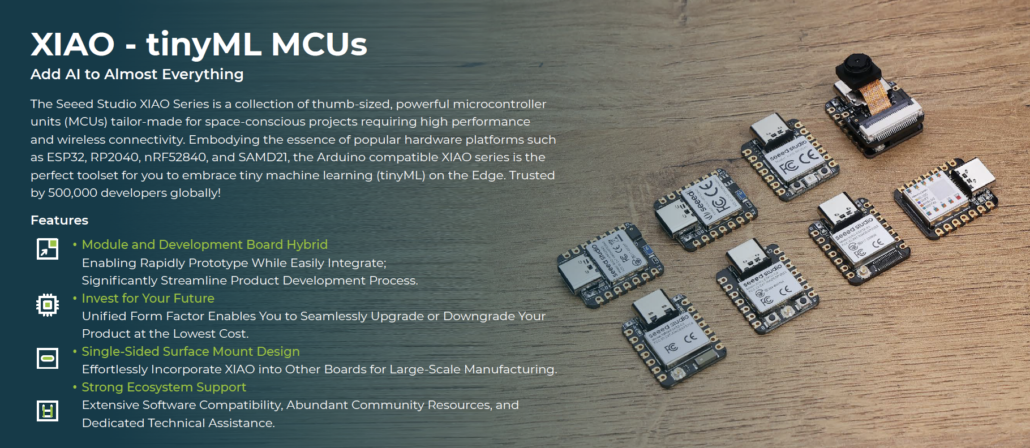
Edge Computers
The representative products in this category are the reComputer Nvidia Jetson series. These are capable of running local Generative AI (Gen AI), ranging from the entry-level 40 TOPS J3011 to the high-performance 275 TOPS J50.
Click here to learn more about this product line for Gen AI.
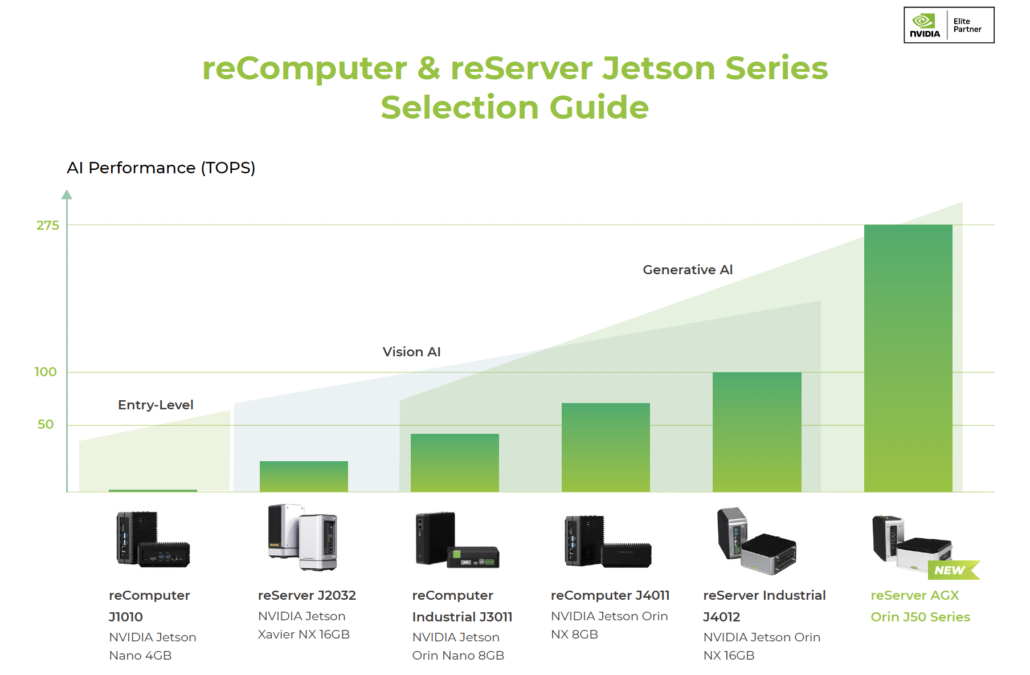
I hope the insights and information shared have been enlightening and inspiring.
If you have any thoughts, experiences, or questions you’d like to share, please don’t hesitate to comment below.